The recent book “Why Machines Will Never Rule the World: Artificial Intelligence without Fear” by Jobst Landgrebe and Barry Smith—amongst the world's most influential living ontologists—provides a timely reminder of the fundamental limits of AI.
At the core of the book is an argument about the nature of intelligence: that for all the remarkable capabilities of machines today, there exists no machine—nor even any known mathematics—that can begin to approach the characteristics or capabilities of human intelligence.
Sophisticated capabilities, simple machines
The publication of this book is especially timely in the context of the recent remarkable advances in the capabilities of large language models (LLMs), most notably ChatGPT.
The ability of LLMs to match or exceed human abilities in a range of writing, drawing, coding, and other tasks has surprised everyone, including their creators. As Stephen Wolfram points out in his excellent explanation of ChatGPT, there is no theory behind these capabilities; rather “it’s just a matter of what’s been found to work in practice”.
For all their sophisticated capabilities, LLMs such as ChatGPT, BERT, LaMDA, and GPT-4 are at root simply machines for manipulating word frequencies, albeit machines able to draw upon unimaginably vast repositories of human written text. As Virginia Dignum, Professor of Responsible Artificial Intelligence at Umeå University, put it in a recent talk at RMIT University: today’s LLMs are neither “intelligent” (in the sense we would usually associate with the term) nor “artificial” (in the sense that the raw material behind any LLM is human natural language).
No singularity
The Landgrebe-Smith book, however, takes aim at a grander problem than simply LLMs and ChatGPT. The core argument in the book is that no AI today is of a comparable level of complexity to human intelligence, or to even that of higher animals. As a consequence, there can be no AI “singularity”—the hypothesised point in the future where machine intelligence surpasses that of its human creators.
Their argument is founded on the distinction between complex systems, such as climate, unemployment, or the stock exchange; and simple systems, such as temperature sensors, cars, or smartphones. Simple systems have well-defined boundaries, progress towards some equilibrium, and have behaviour that can be predicted using logic and mathematics. Complex systems, by contrast, are continually evolving, never reach a state of equilibrium, and exhibit behaviours that cannot be predicted by any mathematics we possess today.
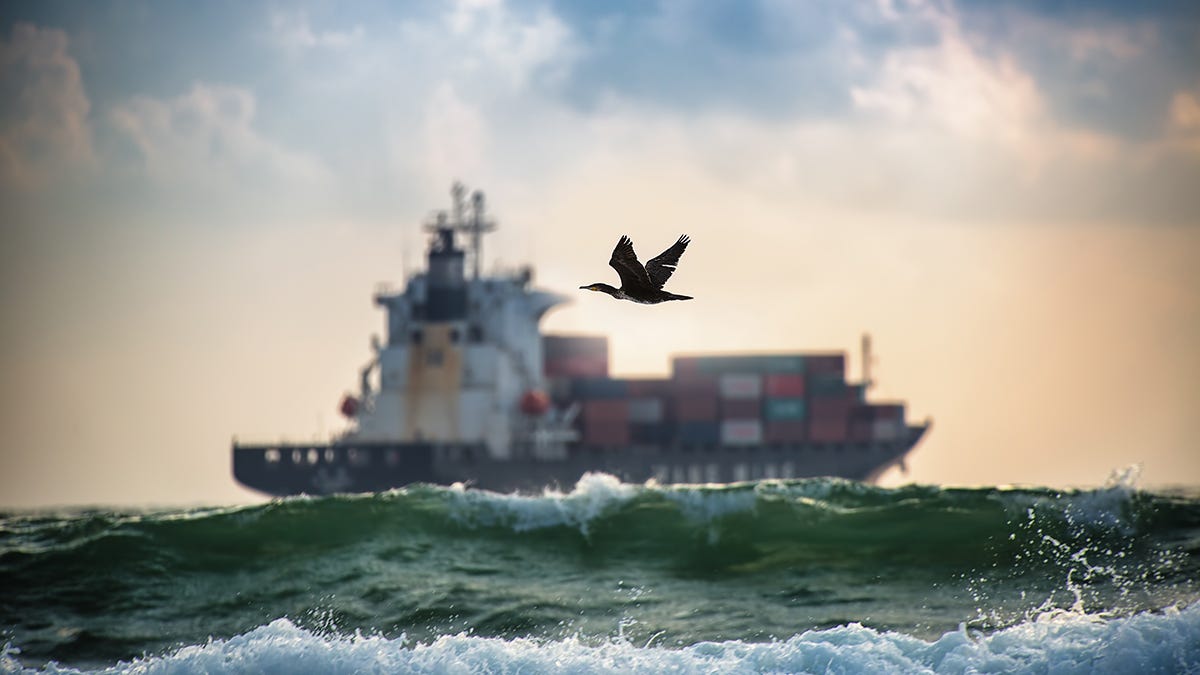
We are able to develop statistical approximations of some complex systems that are useful over the short term, such as those used in weather forecasting. But there is no mathematics, and hence no machine, that can model the behaviour of a complex system, by its very nature. Of course, both simple and complex systems exist together at the same time in the same universe. Distinguishing a simple system, such as the solar system, from a complex system, such as the immune system of a person on a planet in that solar system, is always an act of human fiat. Nevertheless, these two types of system are quite distinct should not be confused with each other.
Human intelligence is a prime example of a complex system. As the book points out, we have today almost no understanding of the nature of human intelligence and how it operates. Aspects of human intelligence can be approximated statistically, but intelligence, thought, and understanding are fundamentally not predictable. The human mind cannot be emulated or even adequately described using today’s mathematics—the mathematics of simple systems. This, Landgrebe and Smith argue, is not just a limitation of AI, but a fundamental limitation of mathematics.
AI with fear
Landgrebe and Smith are certainly not the first to formulate philosophical arguments about the limitations of AI. But the depth and breadth of their treatment is ambitious, and goes beyond contrasting the complexity of human intelligence with the statistical models used in machine learning and AI.
In the book, they also argue that human intelligence is intrinsically connected with our “will” and with human “intention”. Without a will, there can be no autonomy. Without autonomy, AI remains an extension of our own will and intentions. Such machines may make marvellous tools, but cannot possibly rival our own human intelligence.
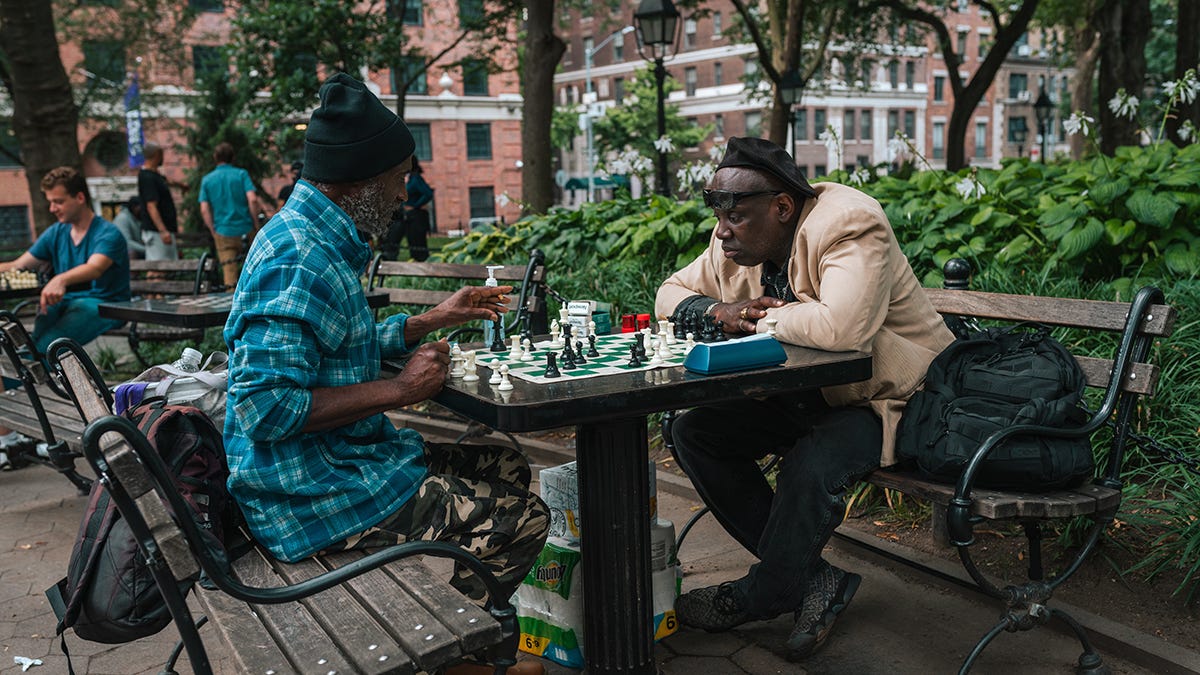
Along this line of reasoning, AI can never have the capacity to start a fight, to be kind, to take up a new hobby, or conceive of a plan to enslave humanity. Machines have no agency, no morals, and cannot exercise judgement or take responsibility. In short, there can be no such thing as an “good” or an “evil” machine.
This is the genesis of the book’s subtitle: “AI without fear”. The responsibility for the use of our marvellous new tools, such as ChatGPT, lies firmly with us, their creators and users, as it always has. But there, indeed, is what we truly have to fear in AI—how we will use it.
Why Machines Will Never Rule the World: Artificial Intelligence without Fear, by Jobst Landgrebe and Barry Smith is published by Routledge.
Share this post