One of the most remarkable impacts of information-based research over recent decades has been the growth in awareness and applications of artificial intelligence (AI) in society. This rise is reflected, for example, in the increasing popularity of searches for information and news related to search terms such as “AI” and “artificial intelligence” (see below, from Google Trends).
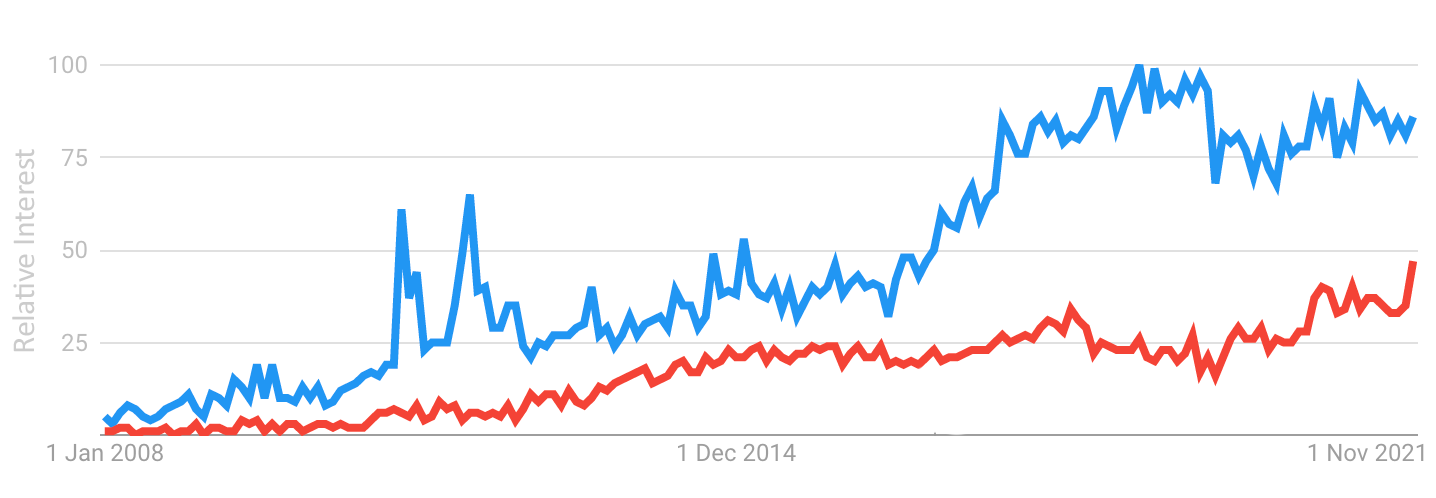
In recent years, much of this growth has been driven by one particular type of AI: machine learning, sometimes also abbreviated to “ML”. Machine learning encompasses a wide range of different techniques. Irrespective of the technical details, all machine learning is fundamentally about automatically identifying patterns in data.
Machine learning and deep learning
Perhaps the most famous of all machine learning techniques today is called “deep learning”. Deep learning has been applied to a dizzying number of new applications over the past decade. Deep learning lies behind the virtual assistance of Siri, Google, and Alexa; the facial recognition used to unlock your phone; the self-driving abilities of autonomous vehicles; as well as many less obvious but everyday applications in fraud detection, healthcare, and even art.
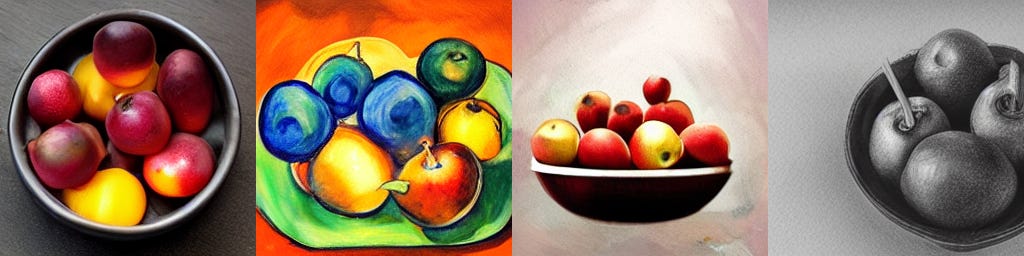
Deep learning itself is founded on a technique called “artificial neural networks” (ANNs). Using the metaphor of biological neurons in the brain as inspiration, artificial neural networks begin with a mass of interconnected artificial neurons. Solving a problem using deep learning first requires conditioning this neural network with examples of existing solutions. The training process progressively reinforces those pathways through the network that on average lead to the correct answer.
The fundamental neural network techniques that underpin deep learning date back to the 1950s. In fact, the rapid growth in deep learning has more to do with advances in raw data processing capacity as in any new algorithms or techniques [1]. It has been the ability to store and process billions of data items for training neural networks that has driven the recent explosion in deep learning.
Once trained, neural networks can perform remarkable feats that rival and in some cases surpass human abilities. For instance, one of the largest neural networks in existence today, GPT-3, was trained using deep learning with billions of examples of human writing across the Web. GPT-3 can generate text that mimics human writing, such as news articles, with uncanny accuracy. As an example, GPT-2 (the predecessor to GPT-3) generated the text below using the first line of this article to seed the machine’s writing as its first line.
One of the most remarkable impacts of information-based research over recent decades is the growth in awareness and applications of AI in society. For example, it seems likely to be that AI will be used to promote social change and the arts, and to help us understand natural sciences, arts and humanities. In other words, if we want to enhance human creativity and to expand our skills, then what should we do now? The fact remains that we don't need to follow the lead set by a few years' or a decade ago. The world of artificial intelligence can and will change, yet we need to understand how the world works and how it functions. Technology and the human mind share a common responsibility.
Knowledge representation
A common limitation of neural networks, deep learning, and indeed machine learning more broadly, is that the machine’s internal mechanisms do not admit a human interpretation of how the machine arrived at its answer. This means it is not possible to say what underlying steps were used to generate the specific text or the images above, for example. A trained neural network is a black box, which leads to difficulties in explaining to a human why exactly the output is the way it is.
In contrast, the second major branch of artificial intelligence, which we can call “knowledge representation” (KR) isn’t subject to this limitation. Instead, knowledge representation aims to encode human knowledge in a machine-readable way, and then use automated reasoning over encoded rules and data to solve problems. Knowledge representation is not as prominent today in the popular consciousness as machine learning. But that has not always been the case. In fact, knowledge representation has historically been the more “famous” branch of artificial intelligence. In 2011, for instance, an IBM machine called Watson, built using knowledge representation techniques, made the news by beating two human champions at the television quiz show Jeopardy.
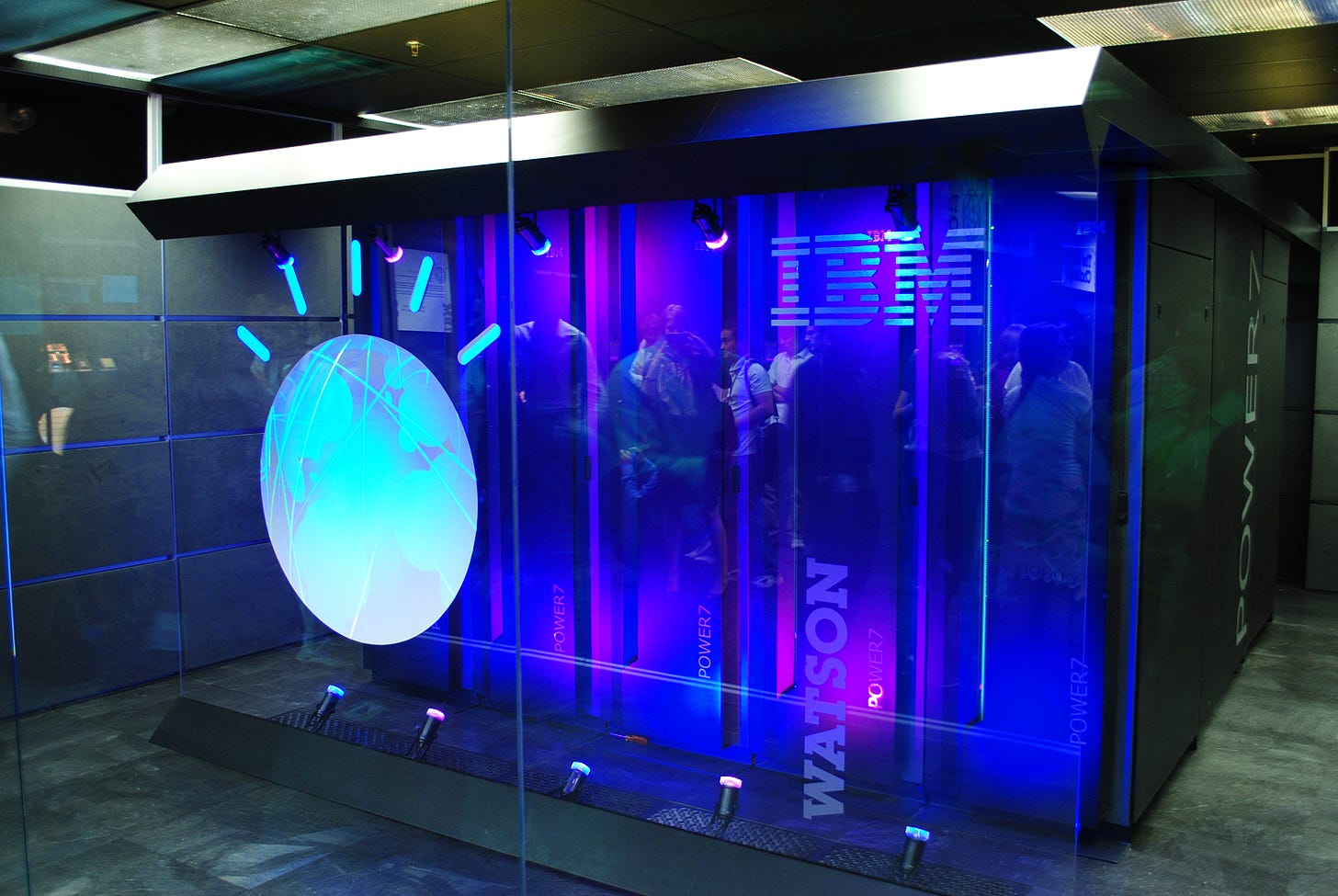
KR’s past glory has been tarnished somewhat by a failure to match over-optimistic expectations in earlier years. Sometimes also referred to as “symbolic AI”, or even pejoratively as “good old-fashioned AI” (GOFAI), Knowledge representation is nevertheless a quiet achiever today, underpinning many important applications of artificial intelligence. Web search engines such as Google rely on knowledge representation and reasoning. A Google search for “What is the capital of Moldova“ correctly returns “Chișinău” thanks to knowledge representation techniques. Similarly, a search for “Paris” or “Melbourne” will bring up a panel with a wealth of information about those cities, such as maps, population, weather, photos, landmarks, and even history, in addition to the regular search results.
These links to related information are made possible not by machine learning, but by a knowledge representation technique called “knowledge graphs”. Knowledge graphs allow us humans to also inspect the mechanisms used by the machines to solve a problem. For example, DBpedia is a knowledge graph of more than 850 million facts constructed from Wikipedia. The DBpedia page for Chișinău, for example, lists hundreds of facts about the city, not only its population, area, and telephone area code; but also that it is the capital of Moldova, the birth place of Olympic table tennis player Sofia Polcanova, and indeed that it is a city.
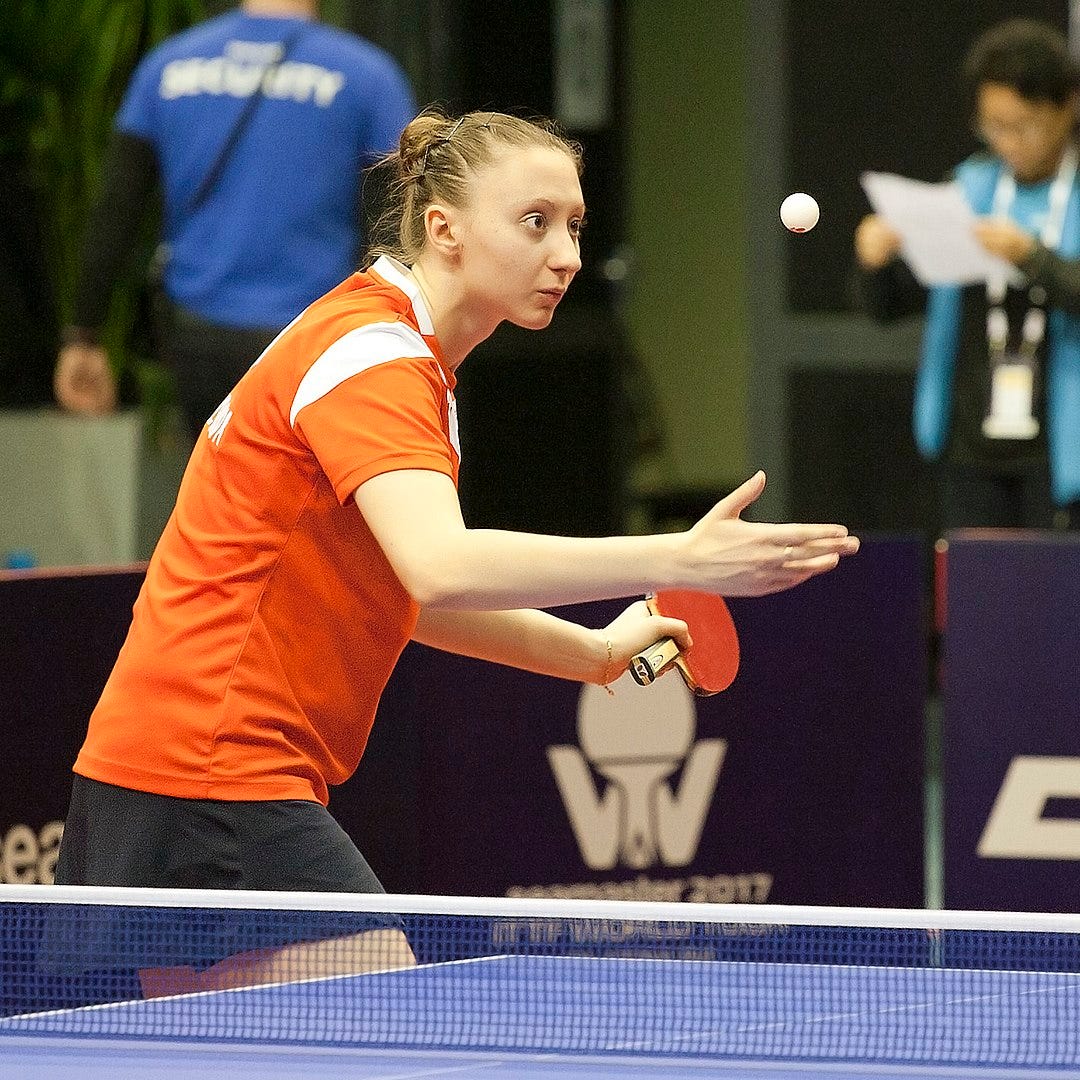
While the DBpedia page is readable as a human, it is constructed directly from a database of simple, machine readable facts, called triples. With a little technical knowledge one can not just retrieve stored data, but ask the machine questions that need logic and reasoning to generate the answer, such as “What’s the largest telecommunication company in Chișinău?”. There exist today many such knowledge graphs, encompassing trillions of facts, all interlinked and all cross-queryable, that power not only Web search, but social media, retail, e-commerce, and news for the world’s biggest companies.
Where next?
Artificial intelligence today remains a very long way from anything approaching human intelligence. Both machine learning and knowledge representation are “brittle”, in the sense that they may perform extremely well in the specific tasks for which they were designed, but typically fail when applied outside that narrow domain. In contrast, humans can manage well in an enormous range of tasks, including applying experiences from one domain to solving new problems they may be encountering for the first ever time.
So for all their apparent smarts, today’s intelligent machines are still pretty dumb by human standards. But on the flip side, that leaves so much still to learn about how to to build intelligent machines. For example, new techniques for combining the capabilities of neural networks to learn from data with the abilities of knowledge representation to reason about human concepts are right now opening exciting new possibilities in the emerging area of “neurosymbolic AI”. So whether machine learning, knowledge representation, or some mix leads to the next big idea, it seems certain that there is much more impact from AI research still to come in the next decade.
References
[1] Thompson, N. C., Greenewald, K., Lee, K., & Manso, G. F. (2020). The computational limits of deep learning. arXiv preprint arXiv:2007.05558.
The rise and rise of AI?